Implementing big team science effectively in economic research
How collaborative approaches can improve transparency, reproducibility and scientific quality
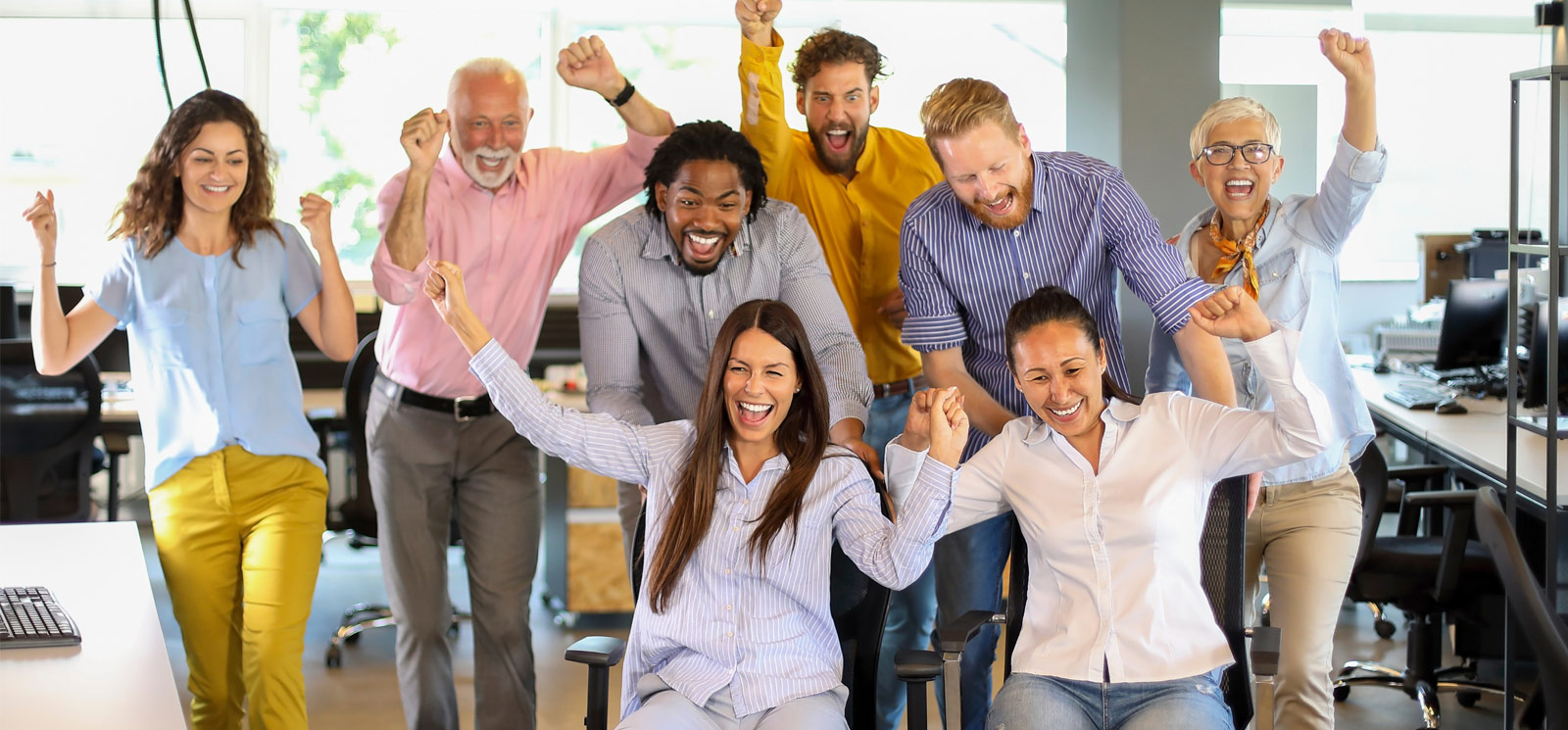
The days when researchers worked on complex scientific questions alone in their laboratories or in a quiet office are increasingly giving way to a new approach: big team science. This collaborative form of science has become established in recent years, primarily as a reaction to the so-called replication crisis, which first became apparent in psychology.
However, big team science is not entirely new: large, international research groups have been working together for decades in the natural sciences, particularly in physics and genetics, to tackle complex issues with enormous amounts of data and computing power. Only recently have the economic sciences also adapted this model in order to improve the methodological transparency, quality and generalisability of their research results.
What is Big Team Science?
Big team science describes comprehensive, collaborative research projects in which several teams or research groups are involved. This form of research offers considerable potential for improving the reproducibility, significance and generalisability of scientific findings and is particularly suitable for dealing with complex economic research issues in a well-founded manner.
Recommended implementation steps:
1. Project coordination and organisation
- Designate clear responsibilities: A coordinating team or individual coordinators take on central tasks such as communication, project management and scheduling.
- Create a detailed project schedule and define binding milestones and clear tasks for each team involved.
2. Standardisation and method control
- Develop uniform standards and protocols for data collection, data processing and analysis procedures.
- Document materials, survey instruments and analysis procedures so that everyone involved can work consistently and without errors.
3. Technical infrastructure and communication
- Use suitable collaborative platforms for data exchange, documentation and communication (e.g. Open Science Framework (OSF), GitHub).
- Encourage regular and transparent communication between the teams involved in order to recognise and resolve misunderstandings at an early stage.
4. Ensure transparency and reproducibility
- Implement pre-registration tools or use registered reports to ensure methodological transparency and reproducibility of results.
- Document and publish all deviations from original plans systematically and comprehensibly.
5. Sustainable documentation of the results and materials
- Create and publish a permanently accessible replication package with materials, codes and data in open repositories (e.g. OSF, Zenodo).
- Provide research artefacts with unique identifiers (DOI) and use open licences (Creative Commons) to ensure long-term use and citability.
6. Dissemination and knowledge exchange across different target groups
- Publish results early via preprint servers (e.g. SSRN, PsyArXiv, EconStor) to integrate feedback from your scientific community.
- Use targeted science communication channels to reach practitioners and a wider audience (e.g. social media, blogs, podcasts).
Benefits of Big Team Science at a glance:
- Improved methodological transparency and increased credibility
- Reduction of publication bias and methodological errors
- Promoting cooperation across disciplinary and institutional boundaries
These systematic recommendations enable the successful implementation of big team science in economic research and contribute to the long-term improvement of scientific knowledge.
References for further reading:
- Deer, L., Adler, S. J., Datta, H., Mizik, N., & Sarstedt, M. (2024). Toward open science in marketing research. International Journal of Research in Marketing. https://doi.org/10.1016/j.ijresmar.2024.12.005
- Duckworth, A. L., & Milkman, K. L. (2022). A guide to megastudies. PNAS nexus, 1(5), pgac214. https://doi.org/10.1093/pnasnexus/pgac214
- Forscher, P. S., Wagenmakers, E. J., Coles, N. A., Silan, M. A., Dutra, N., Basnight-Brown, D., & IJzerman, H. (2023). The benefits, barriers, and risks of big-team science. Perspectives on Psychological Science, 18(3), 607-623. https://doi.org/10.1177/17456916221082970
- Klein, R. A., Ratliff, K. A., Vianello, M., Adams Jr, R. B., Bahník, Š., Bernstein, M. J., … & Nosek, B. A. (2014). Investigating variation in replicability. Social psychology.
- Verschuere, B., Meijer, E. H., Jim, A., Hoogesteyn, K., Orthey, R., McCarthy, R. J., … & Yıldız, E. (2018). Registered replication report on Mazar, Amir, and Ariely (2008). Advances in Methods and Practices in Psychological Science, 1(3), 299-317. https://doi.org/10.1177/2515245918781032.
- Milkman, K. L., Gromet, D., Ho, H., Kay, J. S., Lee, T. W., Pandiloski, P., … & Duckworth, A. L. (2021). Megastudies improve the impact of applied behavioural science. Nature, 600(7889), 478-483. https://doi.org/10.1038/s41586-021-04128-4.
- Sarstedt, M., Adler, S. J., Ringle, C. M., Cho, G., Diamantopoulos, A., Hwang, H., & Liengaard, B. D. (2024). Same model, same data, but different outcomes: Evaluating the impact of method choices in structural equation modelling. Journal of Product Innovation Management, 41(6), 1100-1117. https://doi.org/10.1111/jpim.12738.
*The practical guide was written on 14 March 2025.
This text was translated on 24 March 2025 using DeeplPro.