Efficient open science requires effective data organization
Daniel Evans’ experience of Open Science
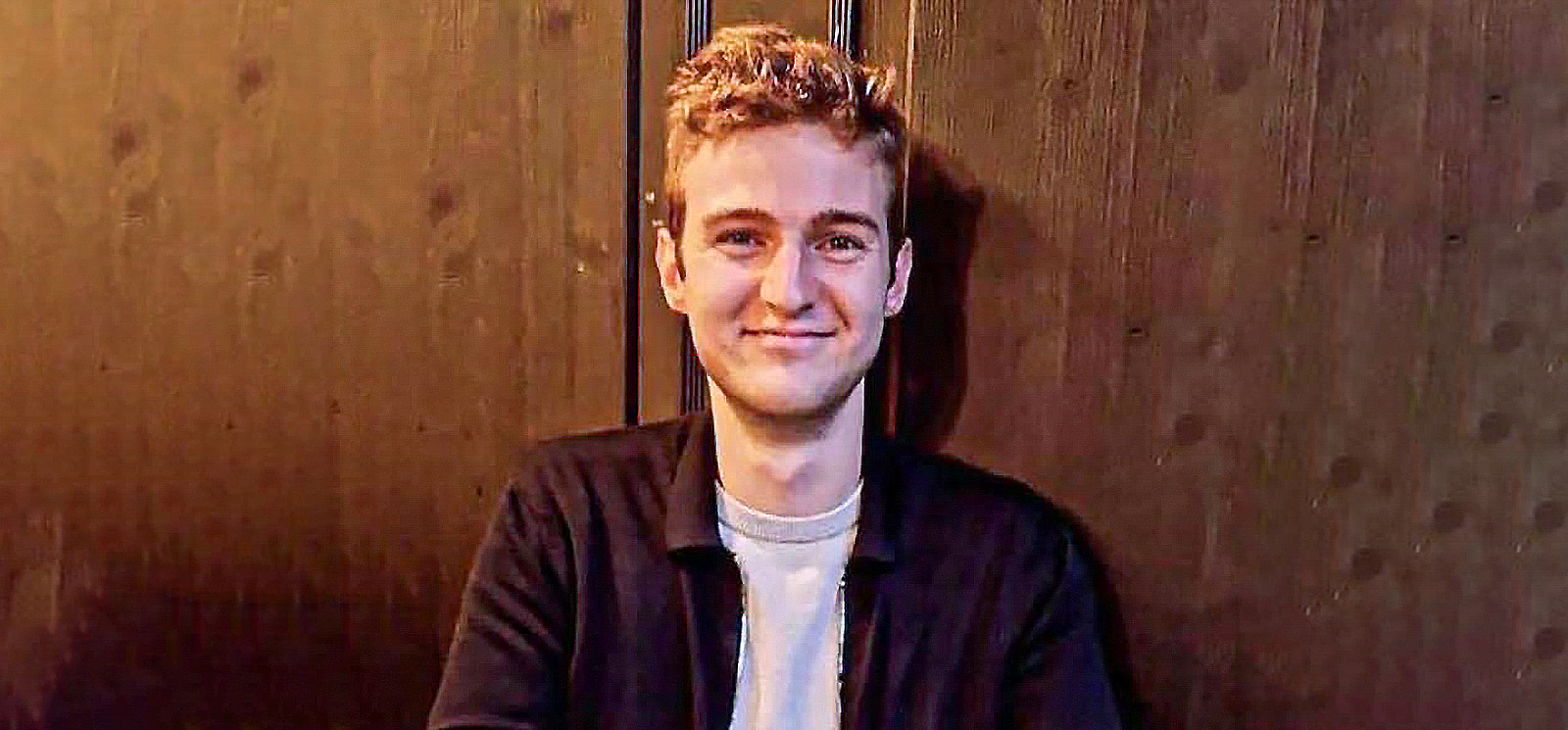
The three key learnings:
- The open science movement contributes to the credibility of research and improves the availability of data and code, which increases the traceability and verifiability of research results. These practices are particularly important for making scientific claims and predictions transparent and verifiable.
- For successful integration of Open Science into research, it is recommended to create reproducible structures for data and analyses from the outset.
- Good organisation and preparation can save time in the long run and improve the quality of research by supporting reproducibility and transparency.
What is your field of research?
DE: I seek to contribute to the literature at the intersection of behavioral economics and two other fields: the science of science (meta-science and innovation) and labor economics. Within behavioral economics, my main focus is on beliefs, with a particular emphasis on the formation, elicitation, and performance of forecasts, i.e., beliefs about future events or variables. Most of my projects explore the implications of biased and/or noisy beliefs for important economic outcomes.
What is your general stance on the topic of Open Science?
DE: My first exposure to Open Science came as a Master’s student at the Stockholm School of Economics. There, I was lucky to meet Anna Dreber and Magnus Johannesson, two enthusiastic proponents of Open Science practices, and to have my Master’s thesis supervised by Magnus. While there, I was recruited by Anna to write a report on the state of the peer review process in economics, jointly with her, Gary Charness, Adam Gill, and Séverine Toussaert. We have one chapter of this report entirely devoted to the (low) prevalence of open access, open reviewing, and other Open Science practices in the peer review process in economics.
On the whole, I think that the Open Science movement has been a net positive for the credibility and usefulness of academic research. Researchers and journals seem more skeptical of novel, flashy-sounding results than they once were, given that many of these eventually fail to replicate. Furthermore, the code and data needed to reproduce manuscripts are now routinely publicly shared at the time of publication, which allows anyone to conduct basic credibility checks on new research and hopefully de-incentivizes fraudulent and unethical research practices. In the long run, these practices should help the credibility and reputation of social scientific research in the eyes of policymakers and the public.
I do see at least one major caveat to this, though. Open Science practices, such as pre-registration, the preparation of data and code for public release, and enhanced documentation requirements, can be incredibly time consuming for authors, editors, and referees. They can effectively serve as a barrier to entry (and to continuation) for under-resourced and junior researchers. Relatedly, they likely contribute to the trends of increasingly-long manuscripts and journal turnaround times, at least in economics. Instead, I believe our academic culture could benefit from encouraging shorter papers, and more frequent replications, rather than expecting any one paper to provide the definitive answer to a given research question. As with anything, this is just a matter of finding the optimal balance between Open Science practices that benefit our credibility as academics and supporting a lively, responsive academic discourse.
Would you like to give us an example of best practice in your field?
DE: As I mentioned earlier, much of my research has focused on beliefs. In line with this, I have become increasingly fascinated with collecting predictions of research results as an Open Science practice, as advocated by Stefano DellaVigna and others – that is, measuring scientists’ beliefs about the likely results of a particular study. This practice is increasingly common in economics, but has seen relatively little take-up in other disciplines.
Open Science practices typically refer to practices that increase the transparency of the research process, or that make research more accessible, like with Open Access journals. The idea behind collecting predictions of research results is to make transparent the priors of the academic community. That is, since authors often claim that their study produces surprising or novel results, one way of putting these claims to the test is to see whether other scientists can predict the results.
To better understand this practice, I am currently drafting a paper on the practice of predicting social science research results, jointly with Taisuke Imai and Séverine Toussaert. The paper will contain both a narrative review explaining the origin of this practice as well as a quantitative meta-analysis of papers that have used it in the past. We hope to deliver clear assessments of the potential benefits and costs of this practice and help practitioners decide whether they would like to incorporate these predictions into their own research.
Have you had any specific experiences that may have surprised you?
DE: I noted earlier the substantial improvements in the credibility of research and the availability of data/code that I attribute to the Open Science movement. Still, some recent experiences have made me realize that we still have a long way to go. In particular, as I’ve been working on the forecasting meta-analysis project, I’ve had to dig through the documentation and replication packages of many papers. While most authors provide the required material, some fall short when it comes to organizing it and writing accessible documentation to assist potential replicators and other users of the packages. Though I understand the substantial time cost that can be involved in setting up these packages, this seems like a potential area of improvement for the community.
Do you have any tips for scientists who have no previous experience of open science and should pay particular attention to when getting started?
DE: I referred earlier to the substantial time costs that certain Open Science practices can entail. In general, it will likely make your life much easier to structure your code and analysis to be reproducible from the start, rather than modifying your code ex-post to meet the requirements once you get to the publication stage. Setting up a nice coding workflow that is portable between projects takes some upfront investment but is likely worthwhile in the long run.
Thank you very much!
About:
Economist Daniel Evans, a member of the BGSE (Bonn Graduate School of Economics) since October 2021, focuses his research on applied microeconomics and behavioural economics. Under the supervision of Thomas Dohmen and Florian Zimmermann, he works on projects aimed at understanding and analysing the behavioural aspects of economic decision-making processes. His scientific approach and research help to reconcile economic models with real human behaviour, making microeconomics more practical.
Contact: https://www.econ.uni-bonn.de/en/department/doctoral-students/daniel-evans
LinkedIn: https://www.linkedin.com/in/danielevanshandels