Excellence in data education
Understanding the future: Expert insights from BERD Academy – An interview with Markus Herklotz
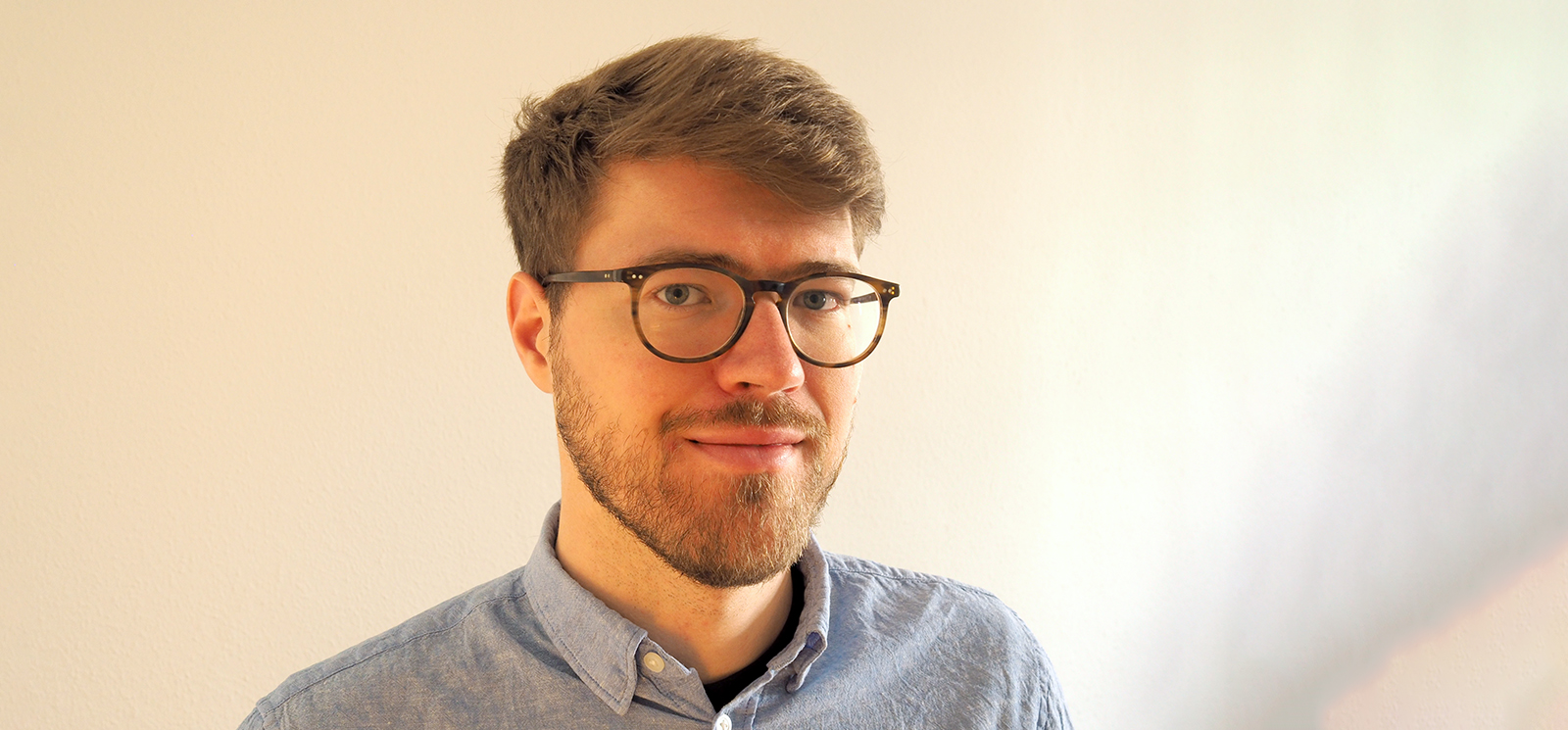
Key Learnings:
- The BERD Academy offers diverse and flexible learning formats for researchers and data specialists in business, economics and social sciences, taking into account their different levels of experience and time resources.
- The courses emphasise the practical applicability of data science and data management concepts in order to facilitate the entry of participants with no previous experience.
- The BERD Academy’s lecturers bring up-to-date and practical content from their areas of expertise, and participants show a high level of engagement once they recognise the relevance of the content to their work.
In an increasingly data-driven world, skills in data science and data management are of supreme value – especially to economic researchers. BERD Academy, an integral part of the BERD@NFDI Initiative, has made it its mission to provide knowledge about data science and data management accessibly and free of cost for economic researchers.
We have spoken with the organiser of the BERD Academy who shares his experiences in a number of fields. We will give insights into the didactic approaches, organisational aspects and the passion for data that drive his work.
Who typically participates in BERD Academy?
MH: In general, we have two groups of participants from the disciplines of business, economics and social sciences: researchers and data service providers (such as data stewards and data librarians). Depending on the topic, the participant profile can span across various experience levels, research interests and job tasks. We address those who already work with unstructured data as well as those who have little experience but want to explore the possibilities of new data types and methods. Curricula at universities are already in the process of changing, but we also invite students to broaden their knowledge with our offers. Some training modules are also designed to bring all levels together.
How do you conceptualise and didactically structure the courses of BERD Academy to achieve your learning goals effectively?
MH: As we primarily target working professionals, one of our main goals is to make the offers compliant with the limited time resources of the participants. Further, participants can have very different learning patterns – some prefer to work autonomously through the material and others like to participate in live settings with a clear schedule. Hence, we try to offer a variety of formats, to accommodate the different necessities. From small coffee lectures over full day workshops to flipped classrooms which run several weeks, everyone should find something to their liking.
What are the proven methods and techniques for successfully teaching people from the domain of economics and social sciences how to code and manage data, especially those who have no previous experience in this field?
MH: One promising approach is to design training as application-oriented as possible. Especially with little time resources, it is more likely to catch participants with something they can directly apply. As research professionals, they have all the tools to further develop these first applications. The first step can be the highest barrier, when they have heard of certain methods or data types, but do not know what they need to learn to reach the necessary skillset. So, we aim to reduce this barrier, providing an interesting entry point and pathways for further development.
When teaching students and researchers from economics and the social science the handling of data, what challenges and pitfalls should be avoided to make their learning voyage more effective?
MH: It is important to acknowledge that they do not start at zero. Most graduates from business, economics and social sciences already bring a valuable toolkit for working with quantitative methods. (Social) data science does not have to be some foreign concept or a completely different discipline but can simply be a broad term for anything data. Hence, more advanced methods may just be an extension of the method kit they are already familiar with. They will very likely have a different approach to data science than, for example, computer scientists, which has to be taken into account. But even within one discipline, the toolkits can be very different based on study program, year, university, and work experience. We therefore offer some bridging introductory courses to specifically address this.
Are there special didactic approaches or teaching materials that have proven particularly effective for giving students and researchers from economics and the social sciences an understanding of data processing and management?
MH: While some concepts of data management at first can seem a bit abstract and researchers might not see the immediate benefit, compared to (for example) concrete analysis methods, they usually have very real-world consequences. So, it is important to not only show the theoretical concepts of, e.g., FAIR data, but what it means in practice. Show them not just what theoretically should be done, but what exactly they can do, and how this improves their work eventually. Fortunately, this topic also gets more recognition because of the valuable work of open science initiatives, the efforts within the national research data infrastructure (NFDI) and other expert groups.
Which courses / topics are most popular with participants??
MH: We have experienced the highest resonance with everything big data and machine learning. This probably relates to the fast developments in these areas, which are not covered in university curricula and require continuous education.
Are there special qualifications or technical requirements for participation in the courses of BERD Academy, in particular the online sessions?
MH: This very much depends on the course. Technically, any device capable of video calls is sufficient for participating online. There are courses which include hands-on programming, so participants will also need a computer and an installation of the (open source) software, usually R. We offer self-paced introductory courses for both R and Python, for which researchers can enroll anytime.
How do you conduct courses on data processing and data analysis at BERD Aademy, especially with regard to Big Data and unstructured data?
MH: In different formats. For example, we have a full day, in-person workshop on network data analysis. Participants are introduced to the theoretical approaches and end the day in a hands-on R lab session, where they work with real-world network data. Another course on microsimulation and machine learning takes place in a flipped classroom format: Participants work every week through the course materials (videos, readings, (R-)assignments) at their own pace and then meet with the instructors and fellow course participants to discuss any questions.
What experience and expert knowledge do instructors of BERD Academy bring to the field of data science and data management?
MH: Our instructors are domain experts from international universities, research institutes, or official statistics institutions. They all bring current topics and state-of-the-art methods to the table and can share valuable practical experiences which the participants as working professionals can relate to. It can be very valuable to not only convey the theoretical knowledge and technical skills, but also to incorporate real-world challenges and to highlight how to use data responsibly.
What’s the experience with researchers from economics and social sciences who must now familiarise themselves with a new domain?
MH: They are usually very motivated which also leads them to find our courses. As mentioned earlier, the highest barrier can be that you first must learn what you need to learn. Once that step is overcome and they see how much of the ‘new’ contents fit very much to what they are already working with, it is much easier to make further progress in the future.
Are there possibilities for self-paced learning at BERD Academy, and how do you organise these courses?
MH: Yes, we offer introductory courses to the programming languages R and Python in a self-paced format. Enrollment in those courses is open permanently. Interested researchers can just register and then receive the access information immediately. We also publish the self-paced part of our flipped classroom courses in various formats, e.g. slides, videos and/or booklets.
How do you sustain the motivation and the interest of students and researchers from economics and the social sciences when it comes to complex technical concepts?
MH: The first step is that the offers are properly framed and every person finds the right level of entry or advanced education. Working professionals usually bring enough intrinsic motivation when they see the benefit of what they chose to learn. Here, I would also suggest that practical application, and thereby showing valuable use cases for making life with data easier, can be a highly motivating factor. In the courses they will also meet other participants, who might have comparable topics and face similar challenges. Some peer effect and exchange which continues even after the course ends, can also be beneficial.
What recommendations would you give to promote the integration of data literacy into the curricula of economics and social sciences or PhD training, and to ensure that graduates have the necessary skills for a modern, data-driven economy?
MH: University curricula are already changing and catching up. Some need for continuing education and lifelong learning comes with this fast-changing field but is also an expected requirement depending on new research insights, just like in any other discipline. The expertise to make advances at universities is already there, through all the excellent researchers engaged in relevant research projects. Some programs are also creating a space for this by incorporating some kind of “current trends in [data science]” courses, where professors or practitioners report directly from new thrilling topics, sparking curiosity on new research opportunities. For that, I like to quote the statistician Chris Wild from a conference this year: “What is the single best thing we can do for our students? To populate their imaginations with possibilities.” Using real-world projects, showing the actual benefits of new methodologies while not ignoring the pitfalls, should be a good set-up for future data scientists from business, economics, and the social sciences.
The interview was conducted on 3 November 2023 by Dr Doreen Siegfried.
About Markus Herklotz:
Markus Herklotz is an education researcher. He works at the BERD project within the framework of the National Research Data Infrastructure where he develops training programmes and educational resources for researchers who handle unstructured economic, business, and related data. His work is focused on helping researchers handle such complex datasets more efficiently.
In his research, Markus Herklotz is interested in the description and assessment of competences in data science as well as the implementation and evaluation of different (e-)learning formats exploring various data sources. He started his academic career in 2015 with a diploma in sociology from TU Dresden.