Meet the international science elite with crowd science
Felix Holzmeister talks about his experience with Open Science
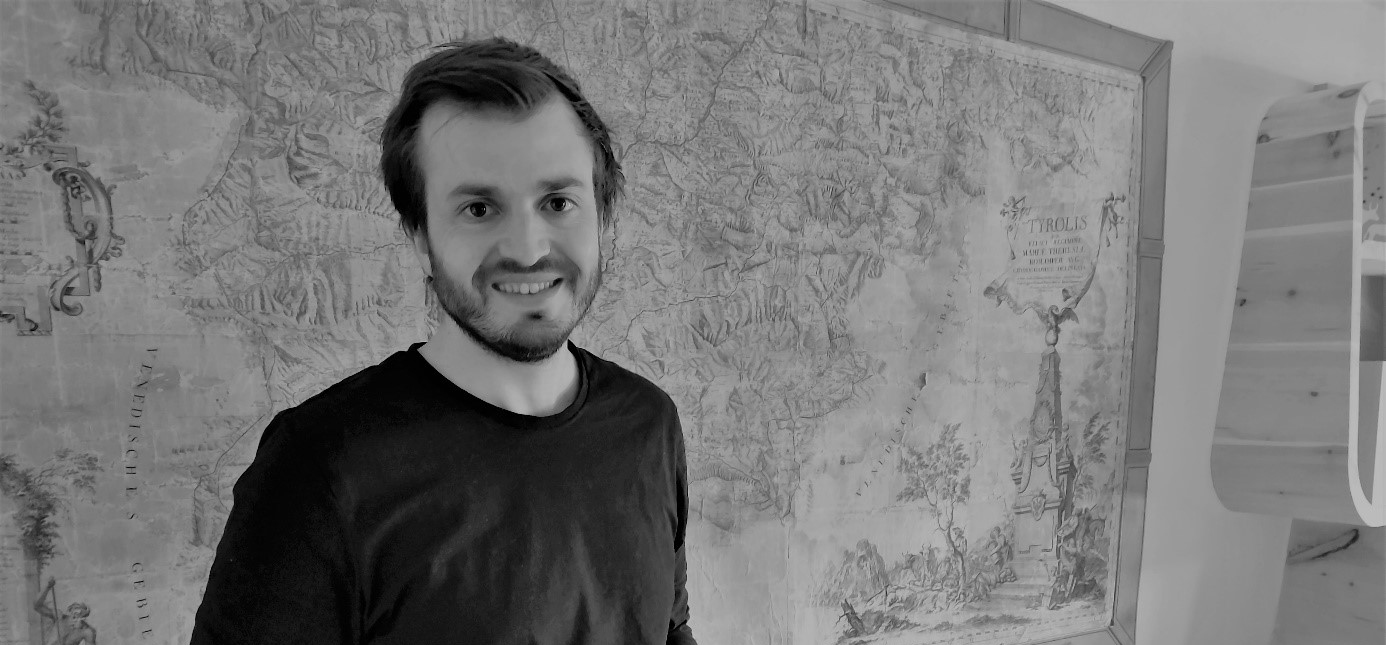
Three key learnings:
- A discipline that wants to be taken seriously needs to examine its own research approaches regularly and critically. This is the basic requirement for a scientific discipline to gain a reputation in the first place.
- Crowd science opens new perspectives on central aspects of the scientific research process and offers new approaches to improving the quality and reliability of research.
- Crowd science helps early career researchers build networks with highly respected peers from all over the world; big team projects can be unique selling points in job interviews.
Since when have you been aware of Open Science?
FH: I got interested when I started my PhD in 2014/15. The first research project in which I actively participated was a large replication study. The topic has been with me ever since. Besides my research in behavourial economics, meta-scientific questions and Open Science have been my second mainstay from the beginning.
Which are the Open Science practices that are particularly relevant to you?
FH: I am a great champion of transparency in every respect. I would even say that transparency – besides the incentive structures in academic practice – are the key for addressing issues that have been raised by meta-scientific studies over the last years. In a first step we should try to sensitise researchers and to convince them that transparency has an essential role in the research process. In a second step we should work to set new – transparent – standards. But this is much more difficult than it appears because the incentive structure in the science system counteracts the process.
What is the subject of your meta-research on replicability?
FH: The first projects have been heavily influenced by Reproducibility Project:Psychology. Basically we did exactly the same thing in our first attempt – only in the field of experimental economic research and on a much smaller scale. We repeated 18 studies which had been published in American Economic Review or in Quarterly Journal of Economics between 2011 and 2014 with as few deviations from the original study as possible. In most cases we worked with the original materials which the original authors generously provided. We observed that only 60 per cent of the research findings could be replicated, and that the magnitude of effects in replications is distinctly lower on average. Next we ran this a second time with studies published in Science and Nature between 2010 and 2015 and in essence came to the same conclusion. At the end of the day, the replicability of experimental results in the social sciences is the same as tossing a coin. Unfortunately, we are a long way away from a situation where you can put absolute faith in published scientific findings.
What was the response to these studies?
FH: Generally speaking, the response has been positive because colleagues recognise very well that there is a problem which must be addressed. But of course there are opposing voices. Some researchers for instance think that the resources required for replication studies would be better invested into generating new knowledge. Although many researchers welcome large projects in this form, the incentives for doing more replications are still missing. In my opinion, the topic of “replicability” and its relevance is insufficiently known. Among the original authors, the response has been positive on the whole, but some felt they were being put to the test. The important thing is that these large-scale projects are not at all about the replication results for individual papers, but about the overall average replication rates for all studies in the sample. Something that gets a bit overlooked in this context is that replications are subject to the same sources of error as the original studies, even if it’s not necessarily to the same degree.
In your experience, is it one of the effects of such meta-studies that economic research has been looking closer at itself?
FH: Definitely. We have seen a clear change of research practices over the last years. From pre-registrations to study designs, from higher transparency and openness to more Open Access publishing – Open Science has entered research in many respects. Reproducibility Project: Psychology set a ball rolling and has created an awareness within the scholarly community about questionable research practices. A discipline that wants to be taken seriously needs to examine itself regularly and critically. That is the basic requirement for a scientific discipline to gain a reputation in the first place. The self-correcting process is a crucial foundation of science. Of course, self-criticism needs to be acceptable for this.
Do you look at other aspects in meta-research besides replicability?
FH: Yes, we have implemented several crowd science projects over the last years – studies where up to 350 authors are involved in a single research project. For example, we have carried out two of the biggest Many Analysts studies ever to find out how large the heterogeneity of scientific findings can be. In both projects we invited researchers from all over the world to test the same hypotheses we stated, based on the same dataset.
How many analysis paths were taken for this?
FH: Almost 80 teams were involved in the first study, and none of them chose the same path to achieve a result. The results and conclusions varied accordingly. We asked the teams in our project to address the same nine hypotheses based on the same dataset. There was a wide consensus for four of these hypotheses: only ten per cent of the teams deviated from the majority in their conclusion. For the other five hypotheses, the uncertainty proved much larger; there was huge disagreement between the teams whether to dismiss the hypotheses or not.
So, despite 80 different analysis paths there has been a robust result for four hypotheses that is rock-solid?
FH: That’s difficult. “Rock-solid” does not exist in the social sciences, on principle. But it is a sign of robustness. However, the robustness of research findings cannot be generalised so easily. This is evident from another study. We recently finished the biggest Many Analysts project so far, based on a dataset with 720 million datapoints provided to us by Deutsche Börse. To our delight we were able to recruit 346 collaborators. More than 160 teams tested the same dataset for six hypotheses which we defined. For each of these six hypotheses there are teams who find a significant positive correlation and teams who identify a significant negative correlation. The heterogeneity in empirical results which arises only from different analytical methods can be substantial. The research process includes an enormous amount of variances, be it the operationalisation of variables, the assumption of theoretical models, the aggregation of data, the handling of outliers, the choice of a statistical model, the consideration of control variables, etc. The path from the raw data to the result is so ramified and requires so many decisions that you can produce both significant positive as well as siginificant negative findings from one and the same dataset for the same hypothesis.
Is there a review for the different analysis paths?
FH: We tried to ensure a high quality for all our projects by setting certain criteria for participation, such as familiarity with the subject, relevant education and publishing in the discipline etc. We take great care that only established researchers are involved as analysts. In our Finance Crowd Analysis Project we also had a peer assessment, but more as a feedback than as a formal peer review. That is, during the first phase of the project the teams received the dataset and the hypotheses to be tested, and they had approximately two months’ time to evaluate the data and to summarise the evaluation methodology and the results in a short paper. We had these short papers reviewed by independent experts who rated each individual hypothesis and gave feedback regarding the methodology. This feedback was passed on to the teams who could then revise their results and re-submit them. In the next step, the five most highly-rated short papers were made available to the teams. So the teams received indirect feedback on which methodology and which results were ranked highest by the independent experts. The teams could revise their own analysis again and re-submit their results. The findings from our study indicate that feedback loops can actually contribute to a reduction in the heterogeneity of results. The variability of the final results remains very large, though.
What does science learn from this, other than that there are many paths and results?
FH: On the one hand, the range of variation in the results shows some kind of uncertainty regarding the actual effect that we are interested in and which is overlooked with the usual means of statistical inference. On the other hand, the variability of the results shows how much leeway there is for questionable practices, such as selective reporting of significant results and p-hacking. Scientists work with an incentive structure which rewards positive results more highly than null results. In other words: researchers have an incentive to select precisely those analysis paths which deliver the result they would like to see. The greater the variance and the resulting heterogeneity of findings, the easier it is to find a significant result.
What are good practices to counteract this, in your opinion?
FH: Of course, questionable practices such as p-hacking and HARKing (Hypothesizing After Results are Known) can be prevented by pre-registration of the planned data evaluation. But where pre-registration alone is insufficient is in showing the uncertainty regarding the result. For a pre-registration I usually select one of many, possibly plausible paths. The uncertainty about which findings would result from other plausible analysis paths remains. One option which is growing in popularity are so-called Multiverse Analyses or Specification Curve Analyses. For this, you choose the essential decision points of an analysis path and for each of these points you define possible plausible decisions. Then you combine all these possibilities factorially, so that a multiverse of paths opens up. Instead of selecting a single, more or less arbitrary analysis path, you analyse all the paths within this multiverse and achieve not only one, but many possible results – a range of results. In this way you can clearly describe the sensitivity of the conclusion to certain analytical decisions.
What kind of feedback do you get for such large-scale projects?
FH: The feedback is mostly very positive. Researchers usually find it very exciting to have a critical mirror held up in front of them. Of course there are also critical voices – and that’s a good thing. Meta-scientific contributions also need to be assessed in nuanced ways to drive further developments.
The approach in itself is quite innovative, as you said yourself, and still far from the mainstream. Have you benefited from this work when you mentioned it in job interviews or other situations?
FH: Yes. I owe my current position to my contributions to the research in this field. But not every university is open and welcoming to crowd science, because the evaluability is different from the usual way of doing things. How do you evaluate the individual contribution of a researcher in a collective of more than one hundred authors? Since it is still customary in many social science departments to use a points system for publications which includes the number of co-authors, a contribution is often not sufficiently valued at the individual level. So young researchers often have no incentive to join crowd science initiatives. However, the contacts and networks arising from such projects can outweigh the disadvantages.
Did these large projects and your general involvement with the topic change your network?
FH: I “stumbled” into an international network very early on. Around 20 researchers were involved in those two replication projects, and among them were some highly renowned scientists from abroad. This was very inspiring and instructive for me, and also opened doors for long-term cooperations. Actually I have been working continously with the same colleagues – and a strong network has grown from it. Especially for young researchers such an international network is highly valuable. It gives a totally different start into an academic career from the one you would have if your only contacts are two or three co-workers sitting in the office next to you.
Who coordinates the 300 authors in such large-scale projects?
FH: In the case of #fincap it was me, actually. To administer a project with several hundred authors sounds very arduous at first, but in my experience it’s actually much easier to coordinate projects of this kind than teamwork among four or five colleagues. Simply because everything runs on much more organised tracks. From the very beginning there’s a clear definition of who’s responsible for what at which time. All team members work to a strictly defined schedule on clearly defined tasks and responsibilities. Planning such a project usually requires more time, but the handling of the project runs much more efficiently.
Is crowd science a model for the future of the social sciences?
FH: In the social sciences, crowd science is a result of what we have learned from the confidence crisis. We are facing problems such as insufficient statistical power, lack of robustness and generalisability of results, p-hacking etc. On average, many published studies – especially studies with little power – simply are not reliable. A small team of researchers generally won’t be able to master these challenges on their own – simply because they don’t have the resources. But they are very much able to join with others, to lump their available resources together, and to implement larger, more differentiated and more informative projects. Focusing on the really important questions while at the same time increasing the quality and the reliability of research findings – that would be my perfect ideal regarding the development of social science research.
Do role models matter? And if so, who would they be?
FH: Role models are a great idea in principle and there are many figureheads in the Open Science movement who fulfil an exemplary role. The first who comes to my mind is Brian Nosek because he has a prominent role in the Centre for Open Science and has been a pioneer from the beginning, finding a voice for the issue at higher levels. We need established scientists like Nosek who show and exemplify that things can be done differently. But it can still be hard to follow such role models if you are not in a position to act against the standards in your immediate environment.
What would be your recommendation to young researchers based on your own experience?
FH: My strongest recommendation to young researchers is to get involved with large crowd science projects – it’s an exciting, valuable and instructive experience. There are many, many projects all over the world who are actively looking for collaborators with open calls via various distributors. Implementing projects of this kind succeeds only if you have a community. If you’re part of such a community you are a small cog in a movement that can change the entire science system for the better.
Thank you!
Dr Doreen Siegfried conducted the interview on 3 November 2022.
About Felix Holzmeister
Felix Holzmeister, PhD is Assistant Professor for Behavioural and Experimental Economics and Finance at the Department of Economics of Innsbruck University and Lab Manager of Innsbruck EconLab. His research interests include different aspects of behavourial and experimental economics, methodological questions in the context of decisions under risk, and markets for credence goods. Felix Holzmeister also focuses on the replicability of scientific findings in behavourial sciences, epistemology, meta-science and Open Science.
Contact: https://www.holzmeister.biz/
ORCID-ID: https://orcid.org/0000-0001-9606-0427
Twitter: https://twitter.com/flxhlzmstr
ResearchGate: https://www.researchgate.net/profile/Felix-Holzmeister