“We need a whole new kind of infrastructure in business studies”
ZBW is a partner for infrastructure in the economics-related NFDI consortia
In October 2021, the NFDI Consortium for Business, Economic and Related Data (BERD@NFDI) was launched. BERD@NFDI plans to build for its community until 2026 a powerful research data infrastructure for collecting, processing, analysing and preserving business, economic and related data – highly networked in different locations, but accessible via a single point of entry.
BERD@NFDI wants to facilitate the integrated management of algorithms and data along the whole research cycle, with a special focus on unstructured (big) data such as video, image, audio, text or mobile data which reflect the behaviour of users in business contexts.
Professor Klaus Tochtermann describes the ZBW’s contribution to BERD@NFDI: “The ZBW is responsible for the development of infrastructure components for BERD@NFDI which support essential phases in the life cycle of research data. The ZBW contributes its knowledge of research data management and digital information infrastructures which have been developed within various research data projects such as GeRDI.”
Professor Florian Stahl, speaker of BERD@NFDI, explains the importance of unstructured data in business studies:
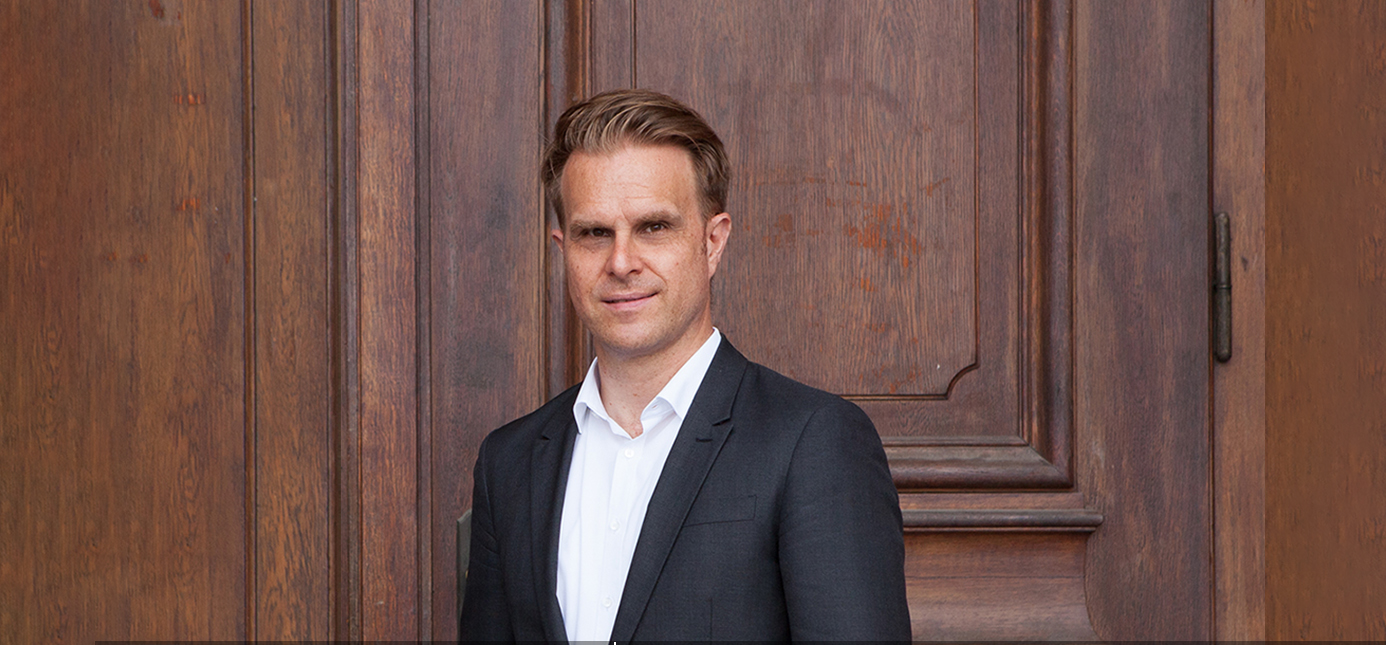
What is the significance of unstructured data in business studies?
FS: There are many intangible topics in business studies, such as brand perception. The brand of a product or a service plays a huge role in business studies. But these can be hard to analyse with quantitative methods if you only have structured data available. Today we have images, text, icons or even videos available in which people express their perception of a brand and their relation to brands. This means that unstructured data offer us completely new opportunities for researching these intangible topics more systematically and quantitatively.
What’s the growth rate of unstructured data?
FS: There are estimates in the terabyte and zettabyte range. The important thing, however, is that the data are growing in diversity and we’re gaining insights into ever more areas of business that have been a black box for researchers until now. The digitisation of all processes in life and business creates data that offer whole new chances for research.
What methods are in use for such data volumes?
FS: In contrast to structured data, unstructured data cannot be evaluated directly with statistical methods. We will have to work heavily with machine learning and other methods of Artificial Intelligence to detect patterns in the unstructured data. With these we can continue our empirical work and statistical analysis.
What is the role of AI methods in business studies?
FS: Over the last five years, Artificial Intelligence has definitely gained hold in all subdisciplines. This is of course related to the new data types, which cannot be evaluated directly without preparatory work. Therefore, AI grows ever more important in the discipline.
What demands does this situation place on a research data infrastructure like BERD?
FS: Business studies is a discipline which is evolving continually and very dynamically. That’s what makes it so attractive. It means that we cannot go on working exclusively with the same methods we were using ten years ago. We must acquire the methods of Artificial Intelligence, of machine learning, and apply them in our research. For this we need a new kind of infrastructure which is not only geared to huge data volumes. We need an infrastructure where we can better exchange and network in the application of methods to certain data types, and of course share resources. That is an essential difference.
Can you name an example?
FS: Yes.If you applya statistical procedure, such as a regression, you always get the same result if you use the same dataset. That’s not the case in Artificial Intelligence, especially in the case of neural networks. For instance, if I train a neural network on Instagram images it is beneficial not only to share the data at the end, but also the neural network. Then, if you want to analyse images from Instagram with another research question some day, it is a benefit for you if you can use not only my Instagram images, but also my neural network. And that’s the difference to past procedures, where you only had to share the data. In the future, we will not only need to archive and share data, we will also need the algorithm which plays an ever bigger role. Otherwise reproducibility is no longer guaranteed.
What are the requirements for such a comprehensive research data infrastructure?
FS: You need storage, computing power, especially for graphics, because different unstructured data must be analysed with different processors. But that’s more an issue of hardware. I think the essential thing is that the discipline must evolve towards Open Science. Right now, many researchers use pre-fabricated solutions provided by large American tech firms, such as Google API or Amazon Web Services. These are quite convenient, but they don’t solve the core problem of saving the algorithm and the neural network together with my data at the end. I’ll say it again: it’s the only way for me to guarantee the reproducibility of my research. Right now, these convenient solutions are very much in use, but in the end they do not meet scientific standards.
Thank you!
The questions were asked by Dr Doreen Siegfried.
BERD – an infrastructure for data, algorithms and neural networks
The focus of BERD@NFDI is not only on data, but also on algorithms and technologies for the collecting, processing and analysing of data. User needs will be identified and taken into consideration by means of close involvement of the learned societies in economics and a survey of early career researchers. BERD@NFDI puts its focus on the integrated management of (un)structured data and corresponding scientific standards in science and business and has a clear commitment to openness (e.g. open software, open standards) and reproducibility (in particular the FAIR Data Principles).
Partners of the ZBW:
- GESIS – Leibniz Institute for the Social Sciences
- Institute for Employment Research
- LMU Munich
- University of Hamburg
- University of Mannheim (coordinator)
- University of Köln