Open Science is a matter of cooperation
Joachim Gassen talks about his experience with Open Science
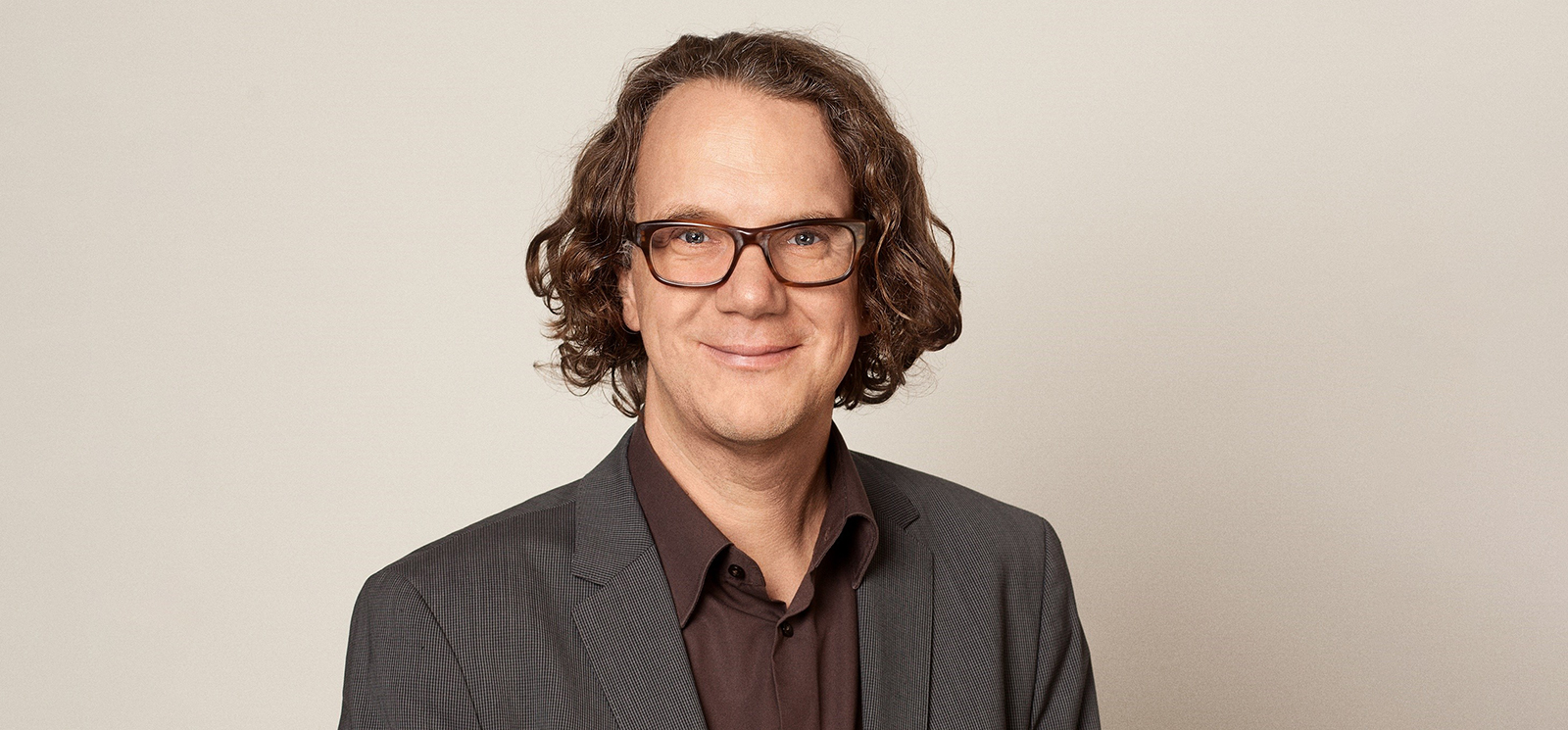
Three key learnings:
- Science needs cooperation because we always build on the methods and findings of others. Progress is better and more enjoyable if it relies on sharing and mutual support.
- Open Science is cooperation. The Open Science workflow is geared to cooperation and thus serves scientific progress. Also: you are always cooperating, even if it’s only with your own future self!
- Learning promotes cooperation. Learning in teams, such as reading, methods, data or coding groups, is perfect for acquiring new skills and starting joint projects.
You head the Open Science Data Centre for the Collaborative Research Centre/Transregio “Accounting for Transparency” at HU Berlin. What exactly do you do at the Open Science Data Centre?
JG: Basically we have three pillars. Our infrastructure services constitute the first pillar. Here we provide the technical data infrastructure for our Transregio and process commercial data, to name an example. This is a work step that doesn’t show in finished papers, but often requires considerable effort. Therefore it makes sense to do this in a central place so that not every scientist has to repeat the same steps.
The second pillar addresses the Open Science workflow. We want to make it easier for the researchers in our Transregio, and in the community in general, to share their data, their code and their methods with others. It starts with conceptual support in matters of “How” and ends operatively with the roll out of data and the curation of datasets, procedures and methods. For this we develop software packages and repository templates supporting these work steps. We also we collect data ourselves from publicly available sources which we administrate and condense so they can be used scientifically.
The third pillar encompasses educational activities in the widest sense and, to be honest, has grown bigger than we expected at the beginning. Here we aim to introduce scientists to Open Science methods. In our experience, problems arise more from the “How” than from the “Whether”. We encounter great openness regarding code sharing and in particular data sharing. But at the same time we also often meet people who are concerned their own work is “not good enough” to be shared publicly. Of course it’s not about the intrinsic quality of the scientific work, but the technical implementation. Scientists always worry that their code is too “messy”. They are also often unsure about data processing and documentation. They are generally much afraid of errors in their own work. With our activities, we want to effect a rethink in two directions: firstly, by adapting a few steps during the development stage, scientific analyses can be made much less error-prone. Secondly, an open and transparent workflow quasi automatically leads to a higher fault tolerance among all involved.
Where do you start in your workshops, coachings etc.? Are there tutorials on “How does GitHub work” or “What do I need to do with Open Science Framework”?
JG: We started with a Statistical Programming Language Course where questions about GitHub and how to use it are important, just like fundamental concepts of functional and object-oriented programming. But the focus is always on practical problems such as “How do I collect data from different sources algorithmically?”, “How do I clean, structure and document them?”, “How do I design reproducible analyses?”, “How do I ensure long-term reproducibility?”, and, increasingly important, “How do I turn my data and methods into ‘products’ that other scientists can use?”. Some of this may sound highly technical, but we try to meet researchers where they are. We don’t want to turn economists into software developers. But we want to convey a few concepts that help being confident in your own data and analysis. This is a crucial point, because researchers are only willing to share their work with others if they feel that it is truly solid. Before the pandemic we had mostly planned face-to-face workshops; they all had to be cancelled, of course. Instead we offered online courses and posted some of our stuff simply on YouTube. Now we see that some of these offers are used by people who don’t work in our field and don’t have a direct relationship with us. We are very happy about that!
Do you cooperate with other universities who also offer Open Science trainings?
JG: Yes, there’s much informal and also formal collaboration at different levels and with different groups. For our online course on Research on Corporate Transparency we collaborated with researchers from many different institutions. Our course on Open Science Methods in Accounting is offered in cooperation with the ProDok programme of the German Academic Association for Business Research. We have also made the experience that role models from their own scientific community can inspire other scientists. That’s not self-evident, because you’d think it makes no difference if it’s a sociologist, political scientist or economist who explains data analysis to me. Ultimately, the workflow is similar even if the data sources vary. But what we actually see is that there’s always a better effect if PhD candidates see someone from their own community making methods, data and codes available. For instance, we have a workflow repository which contains typical working steps of empirical accounting research. It is well received because it offers direct help to accounting researchers. I think decentralised, discipline-specific activities have a lower inhibition threshold, trigger imitation effects, and thus create a real benefit.
Who are the role models in your field?
JG: This may sound a little presumptuous, but I would hope that we provide a certain role model in our small field. Of course we are not the only ones by far. There are other scientists in our field who have always been ahead of the rest. There’s our colleague in the TRR, Laurence van Lent, who has been making data, collated with his co-authors from company publications with methods of Natural Language Processing, available to the general public for quite some time, and he also curates them very carefully. I believe researchers like him set signals and serve as role models. Journal of Accounting Research, one of the leading journals in the field of accounting, is also very pro-active regarding Open Science, and in my view this has also provided a certain thrust. And Lars Vilhuber of course, data editor of the American Economic Association, does a great job in this respect. These initiatives have led us to establish an Open Science track, too, for the Journal of the European Accounting Association, i.e. European Accounting Review.
According to your website, Open Science is a guiding principle for the Transregio. What is it that scientists commit to when they work there?
JG: As a research alliance we have a clear commitment to Open Science, but of course our scientists still enjoy academic freedom. In practice it means that our researchers must give reasons why they are unable to share their materials. And it actually works quite well. Even if not every project lends itself to data and code sharing, we have been successful in ‘nudging’ many researchers towards Open Science. And so it should be. We are a collaborative research centre funded with public money. Our output should therefore be available to the public.
By “mentally reversing” the decision towards “There’s no compulsion, you don’t have to make your materials available, but you need to justify to us if and why you can’t do it”, we have learned a lot about the reasons which, from the researchers’ point of view, speak for or against sharing their materials in the sense of Open Science. As mentioned above, we worried at the beginning that many would give it as their reason that these are their own data which they have collated with much effort and don’t want to share now. But this happened only rarely. Much more often we talked about the timing of making data available. Projects are under review for a notoriously long time, and often researchers don’t want to share their materials before the review process is closed. This is also a question of quality assurance and I can understand that very well. But the reason cited most often is that researchers are unsure how making your materials available works in practice and if they are doing it correctly. And so we’re back to training.
Where do you see particular challenges at the Open Science Data Centre regarding the implementation of Open Science principles? Is it a question of the mindset or of the skills?
JG: Both. Let’s start with the mindset, because I think it’s an important issue in higher education policy. Actually the mindset problem isn’t as big as you hear sometimes. The young generation of researchers have grown up in a knowledge-sharing economy. I don’t know any developer who is not permanently googling and learning things from other people. Which means we have grown accustomed to sharing code, data and software, at least passively. I think the academic labour market, and here very strongly the tenure system, are responsible for creating a mindset problem. As long as code and data sharing and similar activities count for nothing in tenure appointments, we shouldn’t be surprised if early career researchers question the benefit of Open Science for their career. In my opinion this sets perverse incentives and skews the scholarly cooperation model towards a competition model. And there are institutions, universities, associations etc. who explicitly promote this competition. Fortunately, there are also institutions who try to attenuate the idea of competition in science and to emphasise the idea of cooperation. We at the Transregio hope we are among the second group. We are a research alliance and therefore depend on cooperation and we accordingly promote the fundamental idea of “be generous”. It’s my personal conviction that in the long run researchers will have more success and more rewarding careers if they help each other, share and collaborate.
There are numerous other interesting aspects, for example the question of hierarchies within teams: if the PhD candidates in a working group do the majority of the data work and the seniors are mainly responsible for conceptual work and writing up the paper, then the senior co-authors may find it difficult to (co-)decide whether to publish materials or not. On the other hand, the juniors don’t see themselves in a position where they can decide this on their own. I think that says a lot about how we work in teams, or better how we should work. In my view, it’s a problem if true communication about data and methods doesn’t take place within a team and sometimes even can’t happen because of the working processes.
And so we’re back to cooperation. Creating possibilities for cooperation is THE topic in our work. Our claim is “Open Science is all about cooperation”. This even applies to solo projects for which no cooperation is planned, because you can “be kind to your future self: adopt open science methods!” It means that every project you start should be designed for cooperation. Scientific project cycles can be extremely long and when I return to a project on which I last worked a year ago, then I am very grateful to my former self if the steps of the processes are properly documented in a well-configured repository, a good data codebook has been written, and the dependencies in the programming code are documented in ways that make an automated ‘build’ of the project possible. All this requires a certain workflow and certain skills. And that’s the way how Open Science practically embeds itself in the engine room of science.
Where do you see the challenges in arguing for Open Science?
JG: Besides the points we already discussed, one of the biggest challenges in Open Science projects is not only to publish materials but also to ensure that they are actally used. There are vast amounts of scientifically relevant data and algorithms on the web that nobody uses. So it’s essential to offer contributions so interesting and usable that other researchers somehow incorporate them into their research, develop them further and challenge them. In the field of data science they’re also known as “data and code products”.
How do you communicate to stakeholders outside the science system?
JG: In our Transregio we have a communication project where we try to process data in such a way that they are accepted by the public. Once this works you also notice that you need resources for it. For example, we curate a dataset on insolvencies. We process daily insolvency data about German companies and provide them to the public in aggregated form as a dashboard. In the medium term, we want these data to serve primarily for microeconomic and legal research into corporate insolvency. But when we rolled this out to the public the first time, we were hit by a small media wave and by calls from insolvency consultants and lawyers whose interest in the data was commercial. Although public interest is positive in principle, in this particular case a commercial use of the data was not planned for and not possible, for reasons of data protection alone.
Another point that I find enriching is that working in the field of Open Science often means you develop a completely different form of scientific reach, because Open Science issues often have a broader range of application than normal research in the field. In our Transregio we study issues of corporate transparency. But our methods and software packages are used from researchers in totally different fields. The other day I got a very nice email from environmental scientists in the USA who organised a workshop in their lab about one of our software packages. It’s only a small thing, but hugely motivating, I think.
What has been the personal benefit to you of Open Science?
JG: By sharing research materials you develop a different standard for your own output. In this context I have learned so much from other researchers and still do. I am deeply impressed by the productivity of the Scientific Open Source Community. Things that were methodically difficult or (nearly) impossible for intellectually limited people like me are now feasible thanks to the infinite generosity of many. Nowadays I can realise project steps within a week that would have taken me half a year ten years ago. We all use tools as a matter of course today that others have provided altruistically and curate with a lot of their own time. There are others who take the effort of documenting and explaining these tools, so that we can understand them and work with them. And this kind of collaboration is so much more effective than each of us pottering around on their own. Once you have understood this principle of cooperation, you no longer ask yourself what the benefit of Open Science is. It’s obvious.
What options are there for graduates in business studies who are just starting on their PhD thesis to acquire data of software development skills?
JG: On the one hand, there are many good online courses and materials available that make it much easier to familiarise yourself with new methods and procedures. But I do not believe you can become a good data scientist just by googling. You need solid training in statistics and econometrics, but it’s also my conviction that you need motivating learnbing experiences with other people. When I carry out a project togteher with others, I always learn one or two new tricks. The pleasure of discovering something jointly with others, something that nobody knew before and that you can’t google, is hard to top. If I could make a wish, I would wish that we made an empirical group project a compulsory part of the curriculum for bachelor studies, similar to the practical lab courses in the natural sciences. Students could learn how to work with data, produce informative tables and/or graphs to answer manageable, descriptive questions and thus to generate small findings that the world hasn’t seen before.
What would be your recommendation to early career researchers entering the subject?
JG: Don’t be afraid! It’s the same for everyone. The best thing is to start with smaller projects and to find out where you can make your own small contribution. A contribution to the Open Science community doesn’t have to be always technically demanding and methodically complex. For example: Vincent Arel-Bundock, a Professor of Political Science in Canada, has many fabulous Open Science projects going on. But one of my favourite projects is a small R package that makes it easy to assign uniform identifiers to country data. Anyone who’s ever assigned ISO3c country codes to a dataset of 150 arbitrarily written country names, silently swearing all the time, can quickly understand how useful such a small package can be. Another tip would be: it’s better to share small things soon than big things late or never. Big things will never get finished and even if they do, they are usually very specific and can’t easily be used by others. Small things, such as bita of code that solve a specific problem and that you post on Stackoverflow, can be the start into a beautiful Open Science career. Last point: many working groups have reading groups where they discuss current papers. Why not set up data or coding groups? Groups where you meet and talk about issues around data science and help each other.
Let’s take a look at the future, how do you see the subject evolve?
JG: Open Science is reality, although not to the same degree in all fields. I think the significance of Open Science for scientific progress will increase even more. An important point in this context is the role of Open Science contributions in application and promotion processes. Higher education policy needs to reflect more on this and we as scientists must also change our attitudes. In economics at least we reduce research output to journal publications. I believe that’s already an anachronism. Someone who has developed and curates a dataset which is used in thousands of papers obviously makes a scientific contribution. It’s not obvious to me why such people should have to justify themselves compared to someone who has published an article in American Economic Review that has been cited ten times in three years. Science generally takes place and communicates today in many channels. Our research evaluation is still very much focused on journal publications as the undisputed main channel. I am optimistic that this will change in the future, although it may require a nudge from higher education policy.
What also matters to me is the “decommercialising” of data, especially in the area of business. Right now we have the paradox situation that companies are legally obliged to provide comprehensive financial data. The obligation and the right to disseminate these data has been transferred by the Federal Ministry of Justice to a private publisher. The consequence is that scientific use of these data is only possible if you buy a commercial licence and the ministry itself must hand over much money to buy back the data of their companies from a commercial data provider. Such commercial property rights inhibit science and are poison for scientific inclusiveness. My hope is that institutions such as the German Data Forum and the newly established National Research Data Infrastructure will increase their activities in this field and cooperate with the legislative powers to develop wise regulations that protect the individual right to informational autonomy on the one hand, but also make the public data stock available for Open Science projects on the other.
Thank you!
Dr Doreen Siegfried asked the questions.
The interview was conducted on March 31, 2022.
About Professor Joachim Gassen
Professor Joachim Gassen has been professor of accounting and auditing at Humboldt University in Berlin since 2006. Since 2019, he has also been Distinguished Affiliated Professor at European School of Management and Technology in Berlin. He is deputy speaker of the transregional Collaborative Research Centre TRR 266 “Accounting for Transparency”. A team of more than 100 researchers studies how accounting and taxation affect the transparency of companies and what the effects on the economy and society are. In this alliance Joachim Gassen heads the Open Science Data Center which aims to contribute with concrete projects to making economic research more transparent, more cooperative and more inclusive. Joachim Gassen offers courses in Open Science methodology for the PhD programmes of the German Academic Association for Business Research.
Contact: https://www.wiwi.hu-berlin.de/de/professuren/bwl/rwuwp/staff/gassen
ORCID-ID: 0000-0003-4364-2911
Twitter: https://twitter.com/JoachimGassen
LinkedIn: https://www.linkedin.com/in/joachim-gassen-b406b1181/
ResearchGate: https://www.researchgate.net/profile/Joachim-Gassen
GitHub: https://github.com/joachim-gassen
Blog: https://joachim-gassen.github.io