The Open Science principles help reduce the probability of false results
Anne-Laure Boulesteix talks about statistics and her experience with Open Science
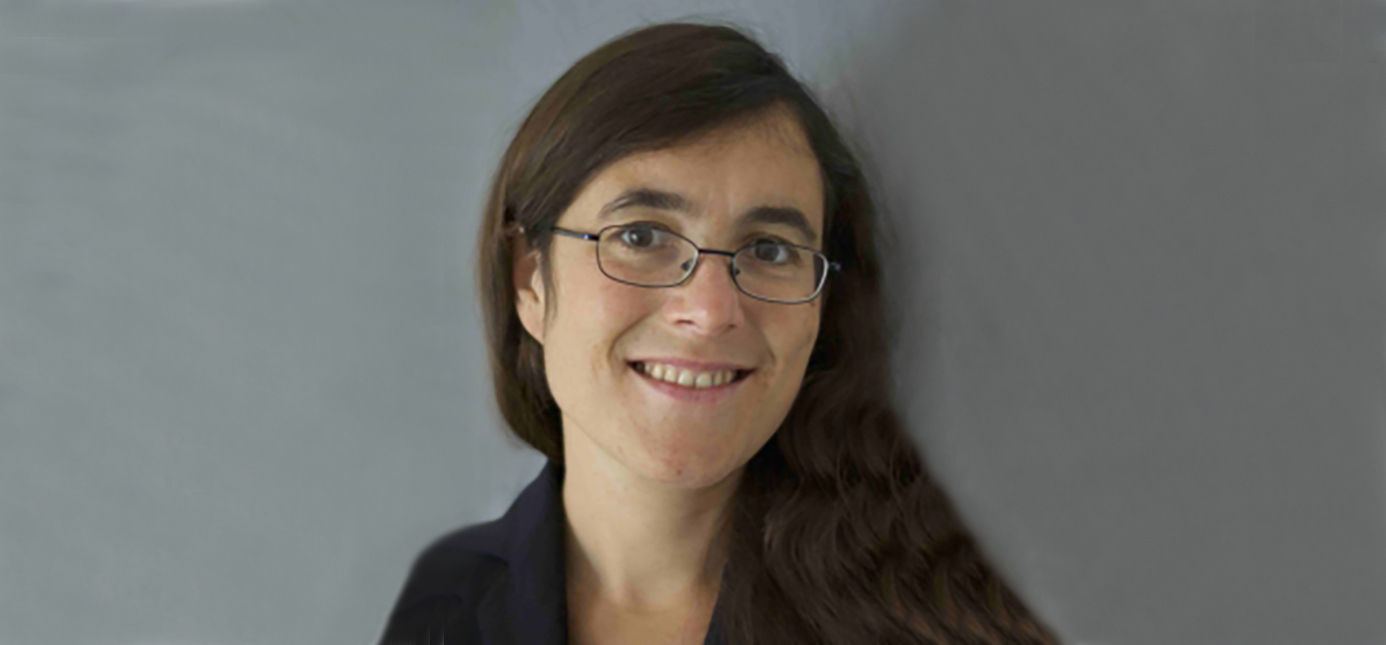
Three key learnings:
- Benchmarking or comparative studies can give guidance for choosing the right method.
- When working with data, microdecisions should best be made result-blindly. It removes the temptation to look for significance.
- A small simulation study resting on the real data can be useful to evaluate the performance of various methods in advance.
What is the probability of published research findings being true?
ALB: This reminds me immediately of the paper “Why Most Published Research Findings Are False” by John Ioannidis. He suggested a kind of model that models the share of true, resp. false results depending on various parameters. The results indicate that the share could be very high. But it is a highly controversial paper. However, given the empirical studies in academic fields which confirm this, I believe that a not insignificant part of published results is false.
How probable this is exactly cannot be put in numbers since it depends on very different parameters. There are fields where many strange hypotheses are tested. This of course leads to a higher risk that hypotheses cannot be confirmed and that the results are false. In other fields the hypotheses are more plausible. Here it is more probable that results are true. But it also depends on how flexible the analysis designs have been. In scientific fields where it is customary to commit in advance to a certain (evaluation) design it is more likely for results to be true. It’s different in fields where it is common to try out many evaluation strategies and then finally to pick the one you like best because the results are pretty. If that happens, there’s a high risk of published results being false. It all depends on the context, and the culture within the discipline, and of course the scientists themselves. Here the Open Science principles can help reduce the probability of false results.
For a few years now, economics has been discussing a replication crisis. How can this crisis be solved? Is it sufficient to make one’s research materials, code, data etc. transparently available?
ALB: Unfortunately there is no universal remedy that can solve all the problems at once. I see this more as a collection of different ideas and principles which can improve the situation gradually in the medium and long term. Nonetheless I can name a few aspects which are important and can contribute to a solution. It’s about reproducibility and replicability, and there is indeed a difference between reproducibility and replicability, although the terminology is not always used consistently in the literature.
Can you briefly explain the difference between reproducibility and replicability?
ALB: Reproducibility means that the authors of a study make the code and the data available so that you repeat all the results, including tables, diagrams and everything, at the click of your mouse, down to the last decimal point. There aren’t many means to ensure this, you need to make code and data available in a sustainable manner. In such a way that it still works two years later. Reproducibility is a bit more straightforward in comparison. Replicability on the other hand is about getting similar or compatible results when you repeat the study with different data. You collect data anew and achieve a similar result. It’s not so easily defined and there’s a lot of metascientific papers about it. In colloquial terms I would say the upshot is the same if you repeat the study with different data. It takes various factors to ensure this. There are some aspects that have nothing to do with statistics: for instance, if I do a laboratory study I must follow certain protocols, and if I don’t do this, my findings are not replicable.
What are the statistical aspects?
ALB: There are many approaches to increasing replicability. For example, you can reduce measuring errors through more precise measuring or increasing the number of cases. I think it’s pretty self-evident that this works. Reproducibility also promotes replicability, because the risk of errors can be reduced by making code and data available. This enables other people, or the authors themselves, to discover potential problems even before publication. This in turn increases the quality and gives researchers the opportunity to rectify. That’s why reproducibility is an important step for more replicability. But there are many other aspects, and they are about the multiplicity of analysis strategies. To put it simply, if you “tinker around” with lots of options and then focus on one result which was just on the border of significance, then it is much less likely that this result is replicated. Results that you get this way are in many cases due to random fluctuations. And if they are random fluctuations, you cannot replicate the result. Sometimes this degree of freedom in data analysis is exploited to make the results look prettier than they are. This definitely contributes to lack of replicability and is an important aspect that has a lot to do with statistics.
What can you do to avoid this?
ALB: In some cases you can commit in advance to an analysis strategy and document this in a protocol, maybe pre-register the study. Because then it’s no longer possible to just tinker around. It’s an important option, but not compatible with all types of studies. It’s more difficult in explorative studies. But explorative research should also be possible. Another option is to say that I cannot very well reduce the multiplicity in my study, but I will set it out transparently. So instead of fishing out one result, the study uncovers a whole range of results, so that readers can see that you get different results depending on what you do. Then it’s transparent.
Would you say it’s legitimate to do an explorative pre-study and to cherry-pick, and then to change my hypothesis and to look at this isolated phenomenon with a big dataset, if I do it transparently?
ALB: That’s a healthy compromise. In this case you reduce the multicplicity in a second stage, and in the first stage you allow yourself to try out many things. It would be some kind of internal validation. You would do a first study which is explorative, and then a confirmatory study within the study. You can also do this procedure which you suggest with your own data. You divide your own data. You use one part for the explorative study to generate a hypothesis and to define an analysis strategy, and you use the other part of the data to confirm the hypothesis. There’s a price of course, because then you have a smaller dataset for each of these two “phases”.
What do you think of so-called crowdsourcing approaches? Where you have a research question, a precise research design and then outsource the task to friendly research groups.
ALB: In principle I think this is very interesting and fascinating. The first crowdsourcing experiments that were made contributed a lot to an awareness of the multiplicity of analysis strategies and they showed how irritating it is for science. A crowdsourcing analysis allows a real view of the entire multiplicity. The analyses are much more diverse as if one scientist or one team said “Okay, now I’ll try this or that”. If there are enough teams, it’s possible to get a complete picture of the diversity of possible analysis strategies. Which of course raises the question of how you summarise that. Is the only purpose of the experiment to show, confirm, and raise awareness of this diversity, or do you want to answer the research question? If you want to answer the research question, you should think about how to create a summary from this diversity. Of course there are efforts in this direction, trying to integrate this uncertainty and to converge them in a kind of synthesis.
What other approaches do you suggest?
ALB: Incentive structures are an important matter for more replicability. What is the acceptance of negative research findings like in the community, in journals, graduate schools or even among appointment commissions and funders? That’s a very important point. Becaue there is a reason why we scientists tend to fish for significance. Scientists run into obstacles when they try to publish negative results. In my opinion, this problem ought to be solved both from the top down as well as from the bottom up. Scientists by themselves can improve many things, but not all.
On the one hand, the science systems rewards spectacular findings. On the other we have funders who demand the promotion of Open Science. How do we get out of this social dilemma? What are the paths to solution?
ALB: I’m not a sociologist or a specialist for science systems but I believe things are changing slowly. There’s progress already. It’s slow, but there are encouraging signs regarding the incentive structures. At LMU, for example, every candidate applying for a chair must give a statement on Open Science. They must explain what they do in this area and their attitude towards Open Science. If this is implemented consistently all over Germany, it will be an importat milestone in my opinion. If this worked optimally, it would free early career researchers from this social dilemma. I believe funders can contribute enormously here by declaring openness to be the standard. They can demand much. I see positive trends here, but it is still a long road.
Back to statistics: when I have collected my raw data and want to evaluate them I face many decisions. What is your recommendation for researchers on how to make the right microdecisions?
ALB: First off I would say: use benchmarking or comparative studies, i.e. large-scale studies neutrally designed and offering neutral comparisons, to guide your choice of suitable methods. In medical statistics, for example, we have the Stratos Initiative. This is an initiative of around one hundred medical statisticians worldwide who aim to create such guidance documents, based on empirical evidence. If there were more of such studies, it could also reduce the options a little. I would encourage everyone to look for such studies and to get such external guidance. There will never be a perfect automatic recipe which can replace a statistician. As a statistician you will always have to make context-sensitive decisions. What I would also recommend is to make these decisions result-blindly, e.g. not to look at the p-value or the estimator for the confidence interval, but instead to look at other aspects, e.g. diagnostic plots for regression models. This avoids the temptation of choosing an analytic path because of a low p-value. A result-blind approach is generally very sensible.
In some cases it may be sensible to do a small simulation study resting on the real data you are analysing. Then you can better evaluate the behaviour of different analysis strategies in advance. Then you may notice that the approach is not that good for the data structure that you have. Personally I think it is sensible to admit transparently at the end of the day that there different ways of analysis, and that you show their outcomes. Depending on the area of application this can be perceived as confusing. And it is not an easy path to take. All in all, the paths I’m suggesting here present only small steps that won’t solve the total problem.
When you look at the replication crisis as a statistician: What is the role of Registered Reports? Does the number of Registered Reports grow?
ALB: My impression is that it is growing. Honesty compels me to say that in my active environment awareness of this is rather high. When I think of my Twitter community, these are people who are interested in such topics. But I think in general you can speak of a positive trend here in which psychologists are pathbreakers, of course. Regarding the Registered Report format, you only need to look at the list of journals involved. It’s evident that this is moving from neuro or psycho sciences to other scientific fields. My impression is also that the young generation has an open ear for this. It’s a well-known phenomenon that the older generation is more careful in their approach. After all, what has always been done like this is now being criticised. It’s difficult for some to hear that it didn’t always work well in the past. The young generation is very much more open about this and I think they are a driving force here.
What’s your experience with replications as an instrument of quality control?
ALB: The way the world of science works right now, you need replication studies before a result can be accepted as established fact. Ideally I think we will need fewer replication studies in future, but we are not yet there. I am an advocate of replication studies and would rate them higher so that they are regarded as full-fledged science. At least if they are well motivated. If replication studies are seen as full-fledged science and if they are published, there will be fewer problems.
When you say that you need to blind yourself, would it also be an option to share this blinding among the research team?
ALB: You would have to think very thoroughly about how to implement this; I don’t think this would be easy. But in principle I think very highly of such blinding strategies. There are approaches in methodical research also, about how to interpret benchmark experiments blindedly. Blinding is also important in clinical studies so doctors aren’t influenced by knowing that a patient has received medication A or B.
Do you think it’s really useful to publish all raw data?
ALB: Yes, I believe it would be good thing. But only together with the analysis scripts that lead from the raw data to the processed data. A stranger usually can’t do anything with raw data alone. But in principle I think it makes sense, because there are many degrees of freedom between the raw and the processed data. There are many steps in which errors can be made, where decisions are made that are sometimes arbitrary because there are many options.
Where do you see the future of the Open Science movement?
ALB: I believe national and international networks are important. It’s also important that early career researchers and students are trained accordingly. Which means early enough. And I think it can only work if there are enough multiplicators who really teach it in practice. Networking at the higher level is also important, of course, because it sends a signal and creates funding. It’s important that the two levels communicate, that the Open Science movement doesn’t work in a higher sphere completely without a connection to the base. And vice versa, it’s no use if you have the best courses and education for students and PhD candidates, but the decision-makers don’t pull along.
Thank you!
The questions were asked by Dr Doreen Siegfried.
The interview was conducted on February 18, 2022.
About Professor Anne-Laure Boulesteix
Anne-Laure Boulesteix is professor at the Institute for Medical Information Processing, Biometry, and Epidemiology of LMU Munich. She studied mathematics and general engineering in Paris and Stuttgart (graduation 2001), gained her PhD in statistics at LMU Munich (2005) and held various post-doc positions in the field of medical statistics. In 2009, Anne-Laure Boulesteix was appointed junior professsor and in 2012 professor at the Institute for Medical Information Processing, Biometry, and Epidemiology at LMU. Beside her research interest at the interface between medical statistics, bioinformatics and machine learning focusing on prognostical modeling, she has been active in the field of metascience for more than ten years. She is an elected member of the board at the LMU Open Science Centre, member of the steering committee of the STRATOS Initiative and co-chair of the STRATOS Simulation Panel.
Contact: https://www.ibe.med.uni-muenchen.de/mitarbeiter/professoren/boulesteix/index.html
Twitter: @BoulesteixLaure
LinkedIn: https://de.linkedin.com/in/anne-laure-boulesteix-7b761a15
ResearchGate: https://www.researchgate.net/profile/Anne-Laure-Boulesteix